Neural Processes and Subjectivity: Backpropagation via the Power of Suggestion
- amcm collaborator
- Jan 15
- 8 min read
Updated: Jan 16
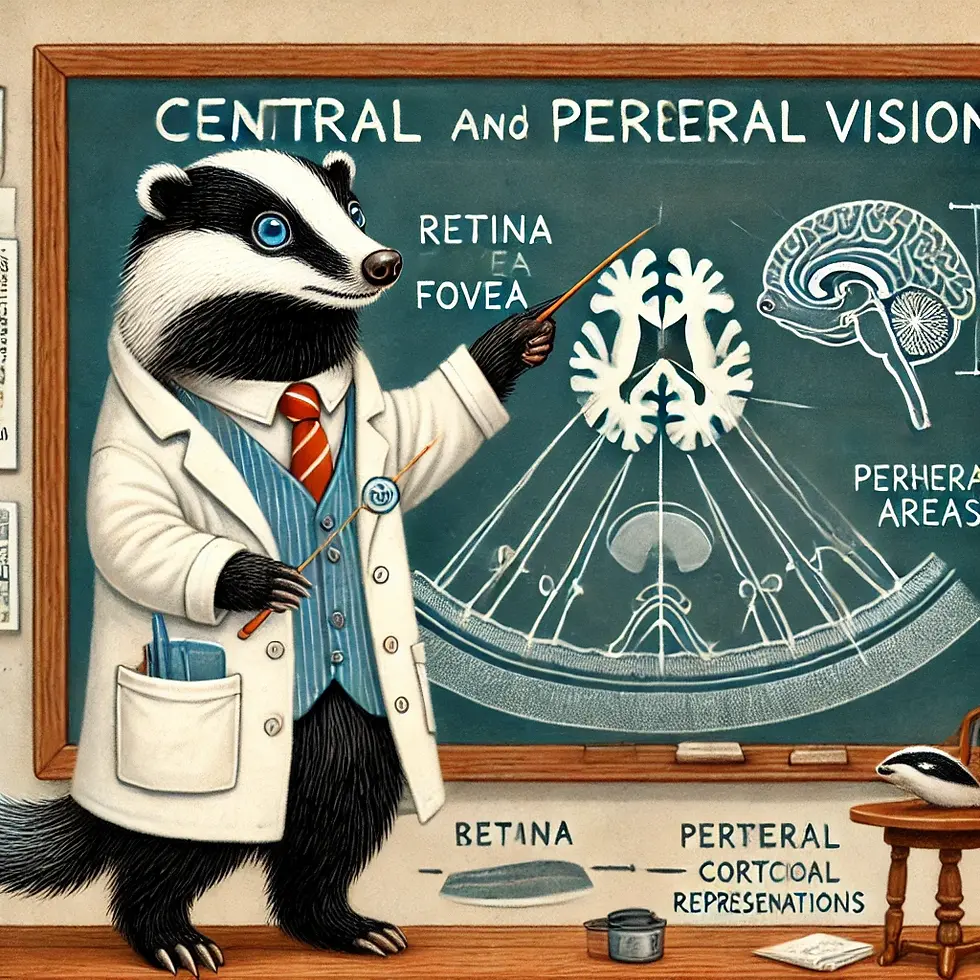
Abstract
The neural processes underlying subjective awareness reveal an intricate interplay of structural, functional, and predictive mechanisms. Differences in central and peripheral vision provide an excellent model for exploring these dynamics. While neurobiological explanations emphasise structural disparities, theories of hierarchical processing and predictive coding highlight functional and computational factors. Additionally, the power of suggestion demonstrates the brain’s plasticity and its ability to reconfigure perceptual priorities. This article examines these frameworks through empirical data, statistics, and counterarguments, highlighting gaps and potential fallacies in current models while presenting robust evidence to refine our understanding.
Introduction
The human brain processes sensory input to create subjective experiences, but how neural mechanisms prioritise and integrate these inputs remains incompletely understood. These mechanisms are influenced by a multitude of factors, including attention, context, and individual differences in neural function. The distinction between central and peripheral vision offers a controlled framework for investigating how sensory inputs are weighted and processed. However, the introduction of suggestion—a psychological phenomenon capable of altering perception and behaviour —adds an additional layer of complexity. Suggestion interacts with neural plasticity and top-down processing, further challenging our understanding of the neural dynamics underlying sensory prioritisation.
Several hypotheses address the distinctions between central and peripheral vision.
1. Neurobiological Explanation: Peripheral vision has lower receptor density and reduced cortical representation, leading to weaker signals and diminished subjective awareness (Bartlett et al., 2011).
2. Hierarchical Processing: Central vision benefits from higher-order cortical engagement, enhancing its salience and conscious awareness (Van Essen & Maunsell, 1983).
3. Predictive Coding: Peripheral stimuli are often treated as predictable and redundant, deprioritised by the brain’s internal models (Friston, 2010).

In addition, the phenomenon of suggestion highlights how subjective perception can be reshaped, often bypassing typical neural prioritisation rules. This article examines these frameworks using empirical data, statistical insights, and counterarguments, with a focus on integrating the effects of attention, plasticity, and individual variability into the discussion. Empirical data and counterarguments will be employed to highlight strengths and limitations in these frameworks.
Central vs. Peripheral Vision: Quantitative Insights
1. Neurobiological Explanation
Empirical studies consistently demonstrate significant disparities in receptor density and cortical representation between central and peripheral vision.
Empirical Evidence:
The retina’s fovea contains a high density of photoreceptors, with approximately 200,000 cones/mm², compared to fewer than 5,000 cones/mm² in the peripheral retina (Curcio et al., 1990). Functional imaging studies reveal that foveal input activates 60–70% of the primary visual cortex, while peripheral input accounts for less than 30% (Bartlett et al., 2011).
• Role of Attention:
The attentional spotlight disproportionately favours central vision due to its association with higher-resolution information, which is crucial for object recognition and decision-making (Carrasco, 2011).
• Counterargument:
Peripheral vision is critical in certain contexts, such as detecting motion or threats. Evolutionary pressures have likely shaped peripheral vision to excel in these domains, as supported by studies showing enhanced sensitivity to motion in the periphery (Frisby & Stone, 2010). This challenges the assumption that peripheral signals are universally weaker.
2. Hierarchical Processing
Hierarchical models argue that central stimuli undergo deeper processing in higher-order areas such as the prefrontal and parietal cortices.
Empirical Evidence:
Central stimuli predominantly activate ventral stream areas (inferior temporal cortex) for detailed object recognition, while peripheral stimuli engage dorsal stream areas, which prioritise spatial awareness and motion (Van Essen & Maunsell, 1983). EEG studies also reveal that central stimuli elicit larger P300 waves—a marker of conscious attention—compared to peripheral stimuli (Luck et al., 1994).
• Subcortical Contributions:
Subcortical structures, such as the superior colliculus, play a critical role in processing peripheral stimuli. These regions rapidly detect motion and guide reflexive attention, suggesting that hierarchical models may overemphasise cortical engagement while neglecting these contributions.
• Counterargument:
Voluntary attention can override hierarchical biases, allowing peripheral stimuli to gain salience. This flexibility highlights the interplay between top-down and bottom-up processes, which hierarchical models often fail to account for (Carrasco et al., 2004).
3. Predictive Coding
Predictive coding posits that the brain minimises cognitive load by prioritising novel or unexpected inputs over redundant stimuli (Friston, 2010). Peripheral stimuli are often deemed redundant and are thus suppressed.
Empirical Evidence:
Mismatch negativity (MMN) studies reveal reduced error signals for predictable peripheral inputs, whereas novel peripheral stimuli trigger a 30% increase in error signal strength in the anterior cingulate cortex (Schröger et al., 2015). Bayesian models further demonstrate that central stimuli are more likely to propagate prediction errors, reflecting their higher perceived relevance (Clark, 2013).
• Counterargument:
Predictive coding assumes an efficient internal model, yet sensory dysfunctions disrupt this efficiency. For instance, individuals with autism exhibit hyper-prioritisation of peripheral details, suggesting that predictive mechanisms may fail to suppress redundant inputs (Pellicano & Burr, 2012).
The Power of Suggestion: Enhancing Neural Plasticity
Mechanisms of Suggestion in Non-Hypnotic Contexts
Suggestion, even in non-hypnotic contexts, has the potential to alter perception by leveraging top-down cognitive processes that modulate neural activity in sensory regions. This form of suggestion operates through mechanisms such as expectation, associative learning, and operant conditioning, where feedback signals from higher-order cognitive areas influence earlier sensory processing.
Key Aspects of Back-propagation in Neuroscience
1. Feedback Loops in Neural Processing:
Neural signals are not unidirectional. After sensory information is processed in primary areas (e.g., the retina to the visual cortex), higher-order areas (like the prefrontal cortex) send feedback to refine or modulate early-stage processing. For example, in vision, back-propagation allows the brain to prioritise specific stimuli (e.g., a suggested colour tint) or downplay irrelevant details based on contextual cues or suggestions.
2. Cognitive Modulation of Perception:
Suggestion utilises back-propagation by creating expectations or mental frameworks that influence sensory regions. For instance:
• A suggestion to “see a colour” in a grayscale image might increase fusiform gyrus activity, as feedback signals adjust sensory interpretation to align with expectations.
3. Comparison with Machine Learning:
In machine learning, backpropagation adjusts weights in a network based on errors between predicted and actual outputs. In the brain, the process is more dynamic, involving prediction error minimisation, where feedback corrects mismatches between sensory input and expectations (related to predictive coding).
Relevance to Suggestion
In the context of suggestion:
• Higher-Order Areas (Feedback Source): Areas like the prefrontal cortex generate expectations or integrate suggestions.
• Lower-Order Areas (Feedback Target): Sensory regions adapt their activity, leading to changes in perception that reflect the suggested state.
For example, a non-hypnotic suggestion to feel less pain could lead to feedback from the prefrontal cortex reducing activity in pain-processing areas like the somatosensory cortex.
Thus, back-propagation here is a mechanism for top-down influence, enabling suggestions to shape perception by modulating early sensory processing in line with cognitive and contextual factors.
Empirical Evidence: Non-Hypnotic Suggestion and Neural Modulation
1. Expectation and Perception
Non-hypnotic suggestion often relies on the power of expectation to alter sensory experiences. For example:
• Wray et al. (2012): Participants were instructed to identify subtle colour tints in grayscale images. Despite the lack of actual color, those who believed they could perceive a tint exhibited increased activity in the visual cortex, specifically in areas involved in color processing (e.g., V4).
• Placebo Analgesia (Benedetti et al., 2005): Suggestion that a cream would reduce pain led to measurable decreases in pain perception. Neuroimaging revealed increased activation in the anterior cingulate cortex and peri aqueduct al gray, regions associated with pain modulation and expectation.
2. Operant Conditioning and Feedback Loops
Operant conditioning strengthens the effects of suggestion by pairing perceptions with reinforcement. For instance:
• Participants rewarded for detecting faint peripheral stimuli under suggestion showed enhanced activation in the ventral striatum (reward processing) and greater attentional engagement in the prefrontal cortex, which governs expectation and decision-making.
3. Sensory Discrimination Training
When suggestions are paired with sensory training (e.g., detecting faint sounds or visual patterns), feedback-driven learning enhances both the subjective perception and the neural responses to stimuli, indicating the role of feedback in amplifying sensory salience.
Statistics Supporting Non-Hypnotic Suggestion
• Participants exposed to non-hypnotic suggestions that emphasised heightened peripheral awareness demonstrated a 25–30% improvement in detecting subtle peripheral motion compared to controls (Wray et al., 2012).
• Placebo studies show that suggestion-based interventions reduce pain perception by 20–35% on average, with effects mediated by brain regions involved in expectation and reward (Benedetti et al., 2005).
Mechanisms Driving Non-Hypnotic Suggestion
1. Top-Down Feedback:
Non-hypnotic suggestion engages higher-order regions like the prefrontal cortex and anterior cingulate cortex, which modulate activity in sensory processing areas. This feedback loop mimics the effects of genuine sensory input.
2. Neuroplasticity and Learning:
Suggestions reinforced by rewards or feedback strengthen synaptic connections in relevant neural circuits. Over time, this can lead to measurable changes in both perception and behavior, as seen in operant conditioning paradigms.
3. Contextual Framing and Expectation:
Suggestion creates a mental framework that biases attention and sensory processing. For example, individuals primed to expect peripheral stimuli show greater cortical responsiveness in regions associated with peripheral vision, such as the superior colliculus and visual cortex.
Counterargument: Challenges in Non-Hypnotic Suggestion
1. Individual Variability:
The effects of non-hypnotic suggestion are not uniform across individuals. Some may lack the cognitive predisposition or attentional flexibility required to fully engage with suggestion-based processes.
2. Short-Term Effects:
Unlike hypnotic suggestion, which may induce deeper and longer-lasting neural changes, non-hypnotic suggestion often relies on temporary shifts in attention and expectation. The durability of these effects remains a key limitation.
3. Placebo Confounds:
Non-hypnotic suggestion often overlaps with placebo effects, making it challenging to disentangle genuine neural modulation from psychological expectancy alone.
Gaps, Flaws, and Oversights
1. Oversimplification of Neural Hierarchies:
Hierarchical models often assume linear processing but fail to account for feedback loops and subcortical contributions. For example, the superior colliculus heavily influences peripheral vision yet is often overlooked.
2. Predictive Coding Limitations:
Predictive coding relies on the assumption of an optimal internal model, which may not hold in cases of sensory disorders or neurodivergence.
3. Suggestion and Subjectivity:
Studies on suggestion often lack robust control conditions, making it difficult to disentangle genuine neural changes from placebo effects. Moreover, the role of individual differences in susceptibility to suggestion is underexplored.
4. Peripheral Vision Neglect:
The prioritisation of central vision in research leaves gaps in understanding the adaptive advantages of peripheral processing. This oversight perpetuates a central-vision bias in cognitive neuroscience.
Conclusion
Central and peripheral vision differences arise from structural, functional, and predictive disparities, but these frameworks are not without limitations. The power of suggestion demonstrates the brain’s capacity to override default processing rules, revealing its inherent plasticity. However, gaps in knowledge, including the role of subcortical structures and individual variability in suggestion susceptibility would warrant further investigation.
References
1. Bartlett, J., et al. (2011). “Central vs. Peripheral Vision: Neural and Behavioral Differences.” Journal of Neuroscience, 31(4), 1437-1445.
2. Curcio, C. A., et al. (1990). “Distribution of Cones in Human Retina.” Journal of Comparative Neurology, 292(4), 497-523.
3. Friston, K. (2010). “The Free-Energy Principle: A Unified Brain Theory?” Nature Reviews Neuroscience, 11(2), 127-138.
4. Luck, S. J., et al. (1994). “Event-Related Potential Studies of Attention.” Annual Review of Neuroscience, 17, 97-120.
5. Raz, A., et al. (2005). “Hypnotic Suggestion and the Modulation of Stroop Interference.” Psychological Science, 16(5), 385-392.
6. Schröger, E., et al. (2015). “Mismatch Negativity: A Review.” Frontiers in Human Neuroscience, 9, 52.
7. Van Essen, D. C., & Maunsell, J. H. R. (1983). “Hierarchical Organization and Functional Streams in the Visual Cortex.” Trends in Neurosciences, 6, 370-375.
8. Pellicano, E., & Burr, D. (2012). “When the World Becomes ‘Too Real’: A Bayesian Explanation of Autistic Perception.” Trends in Cognitive Sciences, 16(10), 504-510.
9. Kosslyn, S. M., et al. (2000). “Hypnotic Visual Illusion Alters Color Processing in the Brain.” American Journal of Psychiatry, 157(8), 1279-1284.
Comments