Jackson Structures
- amuggs82
- Jan 19
- 4 min read
Updated: Feb 4
A Jackson structure is a concept primarily encountered in queuing theory and operations research, specifically in the study of Jackson networks.
A Jackson network is a type of queueing network where multiple queues are connected, and jobs (or customers) move between them according to certain probabilistic rules.
The structure of a Jackson network is based on certain assumptions, making it possible to analyse the behaviour of the system mathematically using Markov processes.
Who Invented It?
The concept of Jackson networks and their structure was introduced by James R. Jackson in 1957. He developed the theoretical framework for these networks, which became foundational for modern queuing theory.
What Is It Used For?
Jackson structures are used to model and analyse systems where resources are shared, and tasks or customers are processed in sequence.
Key Assumptions of a Jackson Network
1. The system consists of multiple queues.
2. Jobs arrive at each queue according to a Poisson process.
3. Service times at each queue are exponentially distributed.
4. Jobs move between queues or exit the system based on predefined probabilities.
5. Each queue operates independently.
These assumptions make Jackson networks tractable for mathematical analysis using Markov chain techniques.
Simple Diagram of a Jackson Structure
Below is an example of a very simple Jackson network with three queues:
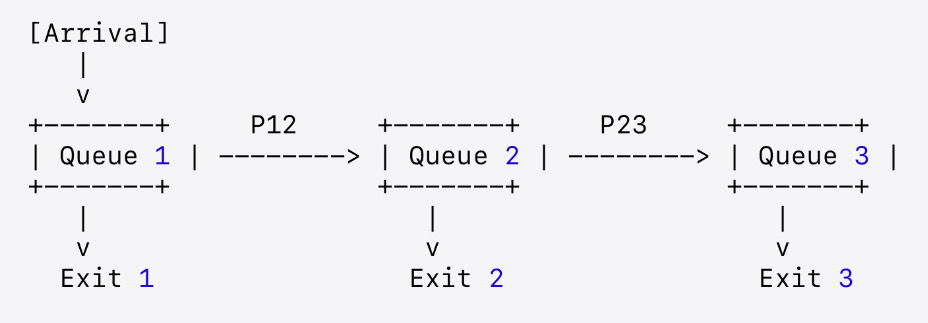
Explanation:
1. Jobs arrive at Queue 1.
2. From Queue 1, jobs move to Queue 2 with probability P12 or they exit the system with probability 1 - P12.
3. Similarly, jobs move from Queue 2 to Queue 3 with probability P23, or exit from Queue 2.
Each queue processes jobs independently, and the flow between queues can be adjusted based on probabilities.
This simplicity and flexibility make Jackson structures invaluable in modelling and optimising complex systems in engineering, business, and logistics.
Behavioural Insights in Health Services
Imagine a speculative scenario where social experiments, cognitive biases, and associative reasoning are strategically leveraged to influence or analyse human behaviour. In the context of health services, such methods might aim to identify individuals in need of potential treatment based on a single variable—let’s call it “X.” This variable could be subtly tied to an association, guiding participants to select specific queues or pathways. The chosen association may unconsciously reflect their preferences, biases, or even underlying health needs, offering a novel and indirect way to assess behaviour and make inferences about their condition.
1. Social Experiments in Queuing Behaviour
Social experiments often study how people behave in public spaces, including how they form queues. Key factors influencing queuing behaviour include:
• Social norms (e.g., lining up in an orderly fashion).
• Cues or signals (e.g., colors, signs, or symbols guiding behavior).
• Group behavior (e.g., following others without questioning why).
2. Use of Fallacies to Elicit Behavior
In this hypothetical application, logical fallacies might be used to influence decisions, such as:
• Bandwagon Fallacy: Individuals might queue where others are queuing, assuming it’s the correct choice.
• Appeal to Authority: A queue marked with an “official” or health-related symbol may attract individuals who associate it with better outcomes.
• False Dichotomy:
Presenting limited options (e.g., Queue A vs. Queue B), when other choices might exist, forces people to make subconscious decisions.
3. Colour Associations and Variable x
Colour psychology suggests that colours can evoke specific emotions or associations:
• Red: Urgency, danger, or importance.
• Blue: Calm, trust, or authority.
• Green: Health, safety, or nature.
• Yellow: Attention, caution, or curiosity.
By associating a colour with a variable x (e.g., a health condition or characteristic), health services might indirectly guide individuals toward specific queues.
For example:
• If green is associated with general wellness and red with critical conditions, individuals may self-sort based on their perceived health status.
4. Practical Implementation in Health Services
Health services might repurpose such methods to:
• Detect subconscious biases: For example, observing who chooses a “green” queue over a “red” one might reveal individuals’ perceived needs or self-assessments.
• Screen candidates for treatment: The queues might lead to different types of care or evaluations, subtly sorting individuals without overtly asking intrusive questions.
• Simplify triage: This method could allow services to streamline patient flow based on minimal input.
Ethical Concerns
While such techniques might offer efficiency, they raise ethical questions:
1. Informed consent: Are individuals aware of how their decisions are being influenced?
2. Bias and fairness: Does this method disadvantage certain groups or reinforce stereotypes?
3. Accuracy: Can a single variable, like x, truly capture a person’s need for treatment?
Diagram Example
Here’s a conceptual diagram of how this might work:

Explanation:
• At a Decision Point, individuals subconsciously choose a queue based on an association tied to variable x.
• Their choice leads them to different types of treatment or further observation.
This hypothetical system merges behavioural science, psychology, and health service optimisation but must be carefully designed to avoid manipulation and ensure equity.
Comments