Financial Markets Integration: Using the Agent in Asset Management
- amuggs82
- Feb 17
- 8 min read
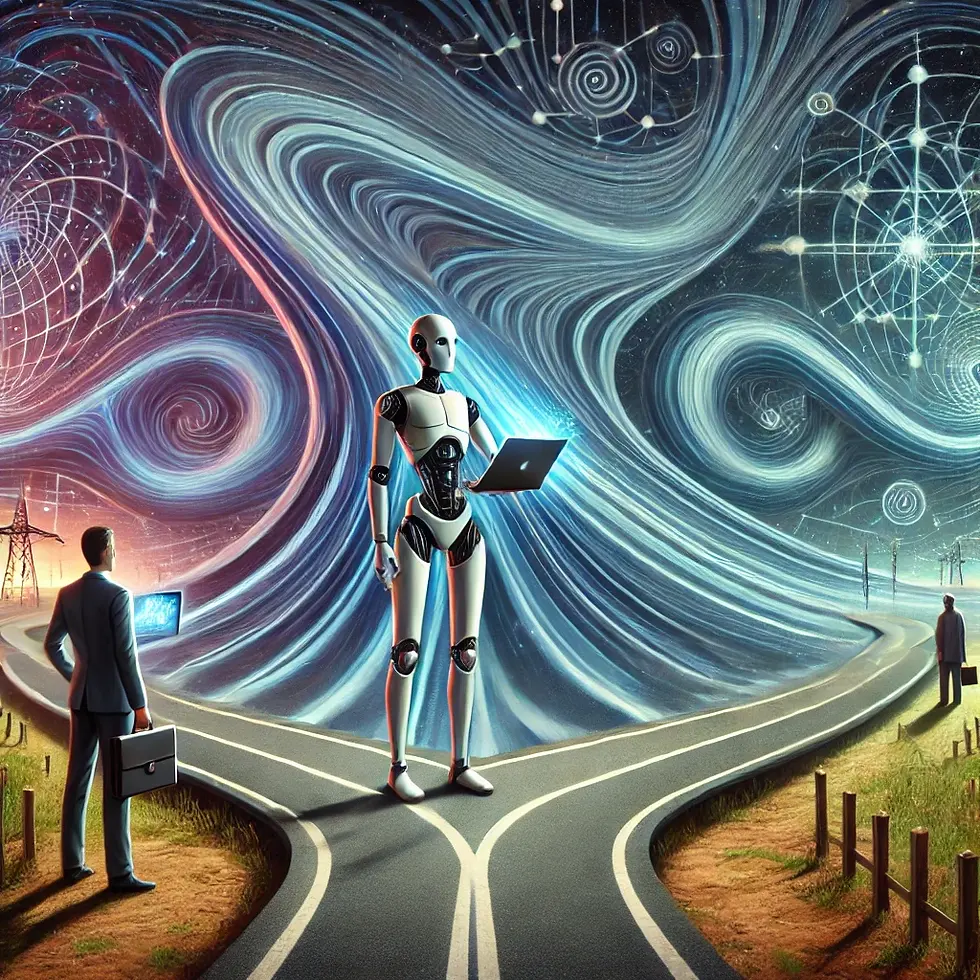
In an era where financial markets are more integrated than ever, the tools that drive market behaviour prediction and portfolio optimisation must be precise, adaptable, and scalable. At the heart of this transformation is the rise of artificial intelligence (AI) and agent-based modeling revolutionary technologies that have allowed financial markets to operate with unprecedented speed and complexity. As an investor, the ability to leverage vast amounts of data, from public financial filings to transaction-level information, to forecast market movements, mitigate risk, and optimise portfolios is the key to remaining ahead in an increasingly competitive global market. Public databases, such as the U.S. Securities and Exchange Commissions EDGAR, provide an essential foundation for financial agents, which can use this data to enhance asset classification and improve market predictions. This paper explores how these cutting-edge technologies are reshaping asset management and unlocking new opportunities for wealth creation.
1. Introduction
As the global market continues to evolve, the ability to harness new technology is the key to capitalising on emerging opportunities. The modern capitalist landscape demands not just market intelligence, but also the precision of AI and machine learning to predict and respond to financial fluctuations. The use of ‘agents’ in asset management is a prime example of this technological revolution, allowing for real-time decision-making and superior asset classification.
Financial agents, empowered by artificial intelligence, have the potential to outperform traditional financial strategies by incorporating vast amounts of data, including detailed financial filings, market analytics, and alternative data. This transformative capability is driven by data repositories such as the SECs EDGAR database, which contains granular, standardised filings from publicly traded companies. These filings such as 10-Ks and 10-Qs are goldmines of information that, when processed by sophisticated financial agents, can predict market behaviour, forecast asset valuations, and ultimately guide investment strategies.
The integration of financial markets is no longer a matter of observing trends; it is about staying ahead of them.
The intersection of AI and agent-based models allows for a level of analysis that can respond instantly to market conditions, whether its a shift in the economy or a sudden geopolitical event. For capitalists who seek to optimise returns and manage risks, these tools are essential.
2. The Role of Agents in Financial Markets
2.1 Defining Financial Agents
The capitalists who understand the power of technology know that financial agents are much more than just algorithms. They are autonomous systems capable of simulating market behavior, identifying trends, and making real-time decisions that can make or break a portfolio. At their core, financial agents use advanced computational models to analyse market data, adjusting investment strategies based on real-time inputs from the market.
These agents operate using different approaches, ranging from reactive to deliberative. Reactive agents are more basic, responding to market stimuli based on preset algorithms or rules. Deliberative agents, on the other hand, are sophisticated, capable of simulating investor decision-making, forecasting future trends, and optimising portfolios based on real-time performance. For the modern capitalist, these deliberative agents represent a new level of competitive edge in an increasingly data-driven world.
2.2 Agent-Based Models (ABMs) in Financial Markets
The power of agent-based models (ABMs) lies in their ability to simulate complex market dynamics. These models don’t just consider individual investors they account for interactions between various agents, creating a broader understanding of how different market participants respond to economic signals, news, and policy changes. ABMs are based on the principles of game theory and complex systems theory, offering a new way to understand the macro and microeconomic forces at play.
ABMs have proven to be particularly valuable in financial markets where behavior is not always rational, and where small changes can cascade into large impacts. Traditional financial models often fail to predict market crises or bubbles, but ABMs driven by a diverse array of agent behaviours can simulate extreme market events, providing invaluable foresight for those who seek to manage risk effectively.
2.3 AI and Machine Learning: The Future of Financial Agents
To fully capitalise on agent-based modeling, AI and machine learning are indispensable. AI-powered agents go beyond simple data processing they learn and adapt. For instance, by analysing financial filings, news articles, and historical market data, agents can detect shifts in market sentiment or forecast the financial standing of companies with far greater accuracy than traditional models.
A key area where AI excels is natural language processing (NLP), which allows agents to analyze the textual content of financial reports such as CEO statements, footnotes, and market commentary extracting sentiment that traditional algorithms miss (Fama & French, 2018). These AI-driven agents can analyse thousands of filings, learning from new data to refine their predictions continuously. For the modern capitalist, this means investments based on predictive insights that evolve in real-time, making traditional analysis methods obsolete.
3. Asset Management Applications
3.1 Asset Classification
The classification of assets is at the core of portfolio management. When one is managing billions in assets, the need for precise asset classification becomes paramount. Agents empowered with data from public repositories, such as EDGAR, can map financial filings to predict an assets behaviour, classify it by risk profile, and optimise its place within a portfolio.
For example, public filings from companies include details about industry sectors, revenue growth, debt ratios, and future outlooks. By processing these filings with advanced machine learning algorithms, agents can assign assets to specific categories growth, value, or high-risk based on both quantitative and qualitative data (Liu & Ravisankar, 2020). These classifications are not static; agents continuously reclassify assets in real-time based on changes in financial standing, market sentiment, or macroeconomic factors, ensuring that portfolios remain adaptable and aligned with current market conditions.
3.1.1 Use of EDGAR Data in Asset Classification
The SECs EDGAR database is a critical resource for financial agents, as it houses standardised and publicly available financial reports for publicly traded companies. By leveraging natural language processing (NLP) and sentiment analysis, agents can process these documents to assess an assets performance and classify it according to risk. For example, an agent might use NLP to detect risk-related language in a company’s quarterly report, such as comments about pending litigation or unfavourable regulatory changes, which could signal a potential decline in stock value.
Furthermore, agents can analyse historical filings to detect trends over time, comparing past financial performance to current reports. This dynamic analysis allows agents to provide more accurate asset classification and performance predictions, vital for the modern capitalist who seeks to maximise returns while minimising exposure to risk.
3.2 Predicting Market Performance
In an interconnected global market, predicting future performance is a delicate science. For the modern capitalist, predicting asset values, stock prices, or even broader market movements based on current data is no longer optional, its a necessity. By integrating financial agents with sophisticated data inputs, investors can accurately forecast market performance in a way that was previously impossible.
Agents do not simply look at one data source they synthesise data from financial reports, stock price movements, and macroeconomic indicators. Regression analysis models are used to identify relationships between market variables and asset prices, while machine learning algorithms continuously refine predictions as new data becomes available (Merton, 1973). These agents also use reinforcement learning techniques to optimise trading strategies, learning from past market behaviour and adjusting to maximise ROI.
By predicting market performance with greater accuracy, financial agents enable investors to optimise portfolios, entering and exiting positions at the most opportune moments. This predictive capability ensures that investments are always aligned with market trends, providing a competitive edge in an increasingly volatile global economy.
3.3 Risk Assessment and Optimisation
Financial markets are inherently risky, but the tools available today enable investors to better assess and mitigate that risk. Financial agents play a key role in stress-testing portfolios against various scenarios be it economic recessions, geopolitical instability, or stock market crashes.
Through advanced portfolio optimisation techniques, agents can handle complex constraints and optimise asset allocation in ways that traditional models cannot. For example, using Markowitzs Mean-Variance Optimisation alongside AI-driven risk assessments, financial agents can create portfolios that offer the best risk-adjusted returns, factoring in real-time market conditions and alternative data such as sentiment analysis or geopolitical events (Markowitz, 1952).
For the modern capitalist, the value of financial agents lies not just in their ability to predict market outcomes but also in their capacity to create a portfolio that is dynamic and resilient. These agents are able to anticipate volatility and adjust holdings to mitigate risks, giving investors the confidence to navigate an uncertain world with precision.
4. Challenges and Future Directions
4.1 Data Quality and Privacy Concerns
As financial agents continue to evolve, concerns about data quality and privacy will become even more critical. The accuracy of predictions depends on the quality of the data used. Public financial filings, while standardised, may contain errors or outdated information. Furthermore, the increasing collection of alternative data such as social media sentiment raises questions about privacy and the ethics of using such data.
As a capitalist at the forefront of these developments, I understand that addressing these challenges requires collaboration with regulatory bodies, investment in better data infrastructure, and a commitment to transparency. While financial agents hold tremendous promise, their effectiveness depends on high-quality, ethical data use.
4.2 Algorithmic Bias and Transparency
Another challenge is the potential for algorithmic bias. If the data used to train financial agents contains inherent biases, it can lead to skewed predictions and unfair outcomes. The transparency of these algorithms is crucial to ensuring that investment decisions are made with a clear understanding of how conclusions are reached. Financial agents must be able to explain their predictions in a way that allows investors to trust their decisions.
For the modern capitalist, the key to overcoming these challenges lies in leading the charge for fairness, transparency, and accountability in AI. By working with regulators and the tech community, we can ensure that financial agents are both accurate and equitable.
5. Conclusion
The integration of financial markets is now more advanced than ever, thanks to the application of AI and agent-based models. As a modern capitalist, the opportunity to leverage these tools for superior asset management and wealth creation is undeniable. Financial agents are not just transforming the way we classify assets or predict market behaviour they are reshaping the future of finance itself.
By tapping into the power of public financial data repositories like EDGAR, financial agents provide a level of insight and precision that traditional models simply cannot match. For those willing to embrace this technology, the rewards are significant: more informed decisions, better risk management, and greater returns. The future of asset management is digital, dynamic, and driven by AI and those who adapt will lead the next generation of capital markets.
“Artificial Intelligence is the new electricity. Just as electricity transformed nearly every industry a century ago, AI is poised to do the same today. It will reshape every field, from healthcare to finance, making all things more intelligent, efficient, and interconnected.” - Andrew Ng, Co-founder of Google Brain and Coursera
References
Angwin, J., Larson, J., Mattu, S., & Kirchner, L. (2016). Machine Bias. ProPublica.
Cohen, L., Diether, K. B., & Malloy, C. J. (2016). Knowing Your Limits: The Role of Information in Asset Allocation Decisions. Review of Financial Studies, 29(8), 2367-2400.
Fama, E. F., & French, K. R. (2018). The Cross-Section of Expected Stock Returns. Journal of Finance, 43(6), 427-465.
Hernandez, L. (2019). Artificial Intelligence in Financial Markets: Cutting Edge Applications for Risk Management, Portfolio Optimization, and Economics. Wiley.
LeBaron, B. (2006). Agent-based computational finance: Suggested readings and early research. Journal of Economic Dynamics and Control, 30(11), 1419-1457.
Liu, W., & Ravisankar, P. (2020). Data Mining in Financial Applications: The Role of AI and Machine Learning in Asset Management. Springer.
Merton, R. C. (1973). Theory of Rational Option Pricing. Bell Journal of Economics, 4(1), 141-183.
Markowitz, H. M. (1952). Portfolio Selection. Journal of Finance, 7(1), 77-91.
Shapira, Z., & Vaish, S. (2019). Reinforcement Learning in Finance: An Overview of Agent-based Approaches for Trading Strategies. Springer.
Tene, O., & Polonetsky, J. (2013). Privacy in the Age of Big Data: A Time for Big Decisions. Stanford Law Review Online, 64(1), 63-69.
Comments